What are the common privacy and security challenges in the era of AI and what are the possible solutions?
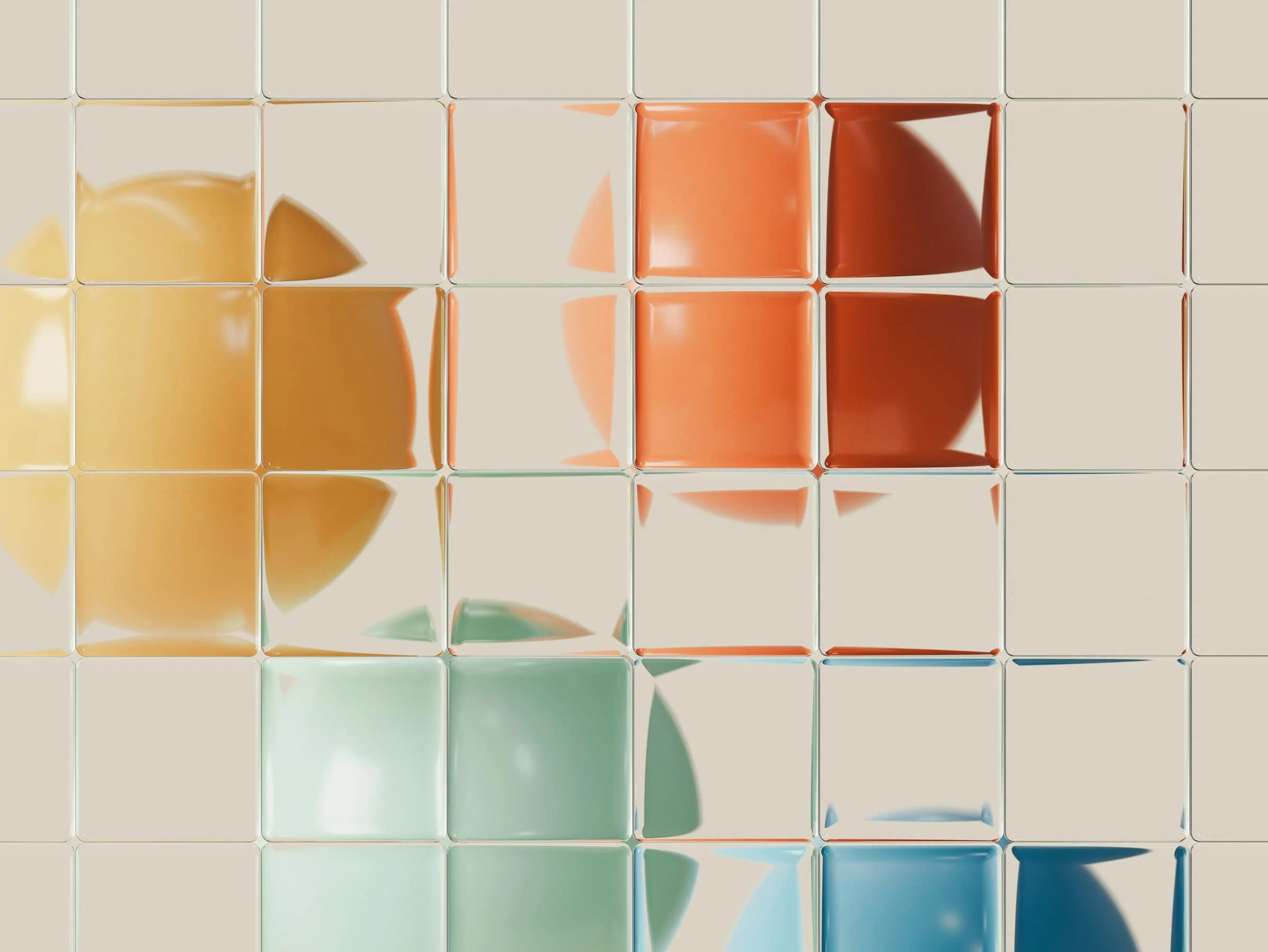
Section 1. Introduction
Artificial Intelligence (AI) is the use of computer programs that have some of the qualities of the human mind, such as the ability to understand language, recognise pictures, and learn from experience (Cambridge Dictionary, 2023). AI is currently widely used in daily life, almost every digital platform people use are powered by AI. While the terms security and privacy are often used interchangeably in literature, it's crucial to emphasize the distinction. Security issues often relate to unauthorised or malicious accesses and modifications that possibly threaten privacy, while privacy issues typically pertain to inadvertent exposure of personally identifiable information, often arising from publicly accessible data (Ma et al., 2020). According to a survey conducted by Ali et al. (2019), one quarter of participants admitted to never having read privacy policy and terms of use and more than half of the people read privacy and policy occasionally. Although it is not clear how many of the participants read the document in its entirety, the data reported appear to support the assumption that only a slight number of people are likely to know how the platforms store and use personal information, as tech companies usually hide the information in Privacy Policy and Terms of Services.
The conflict between preserving privacy and developing digital platforms is not just happening in the era of AI. As a matter of fact, the conflict also happened when the social media started wide spreading. Google uses customers’ searching and viewing history to recommend advertisements, Facebook scan users’ contacts to suggest “new friends”. Though it is assumed that users are all aware of the functions mentioned in their Terms of Services, the lack of readability and user concern has left companies with no boundaries when it comes to digging users’ privacy. Few companies even sometimes directly access users' private information without informing. Facebook, for example, once launched a program called Beacon, which was functioned as a tracker in collecting all kinds of online activities by Facebook users, especially on shopping sites to promote advertisements. What controversial was that without the Facebook user's knowledge, it happened even when users weren't linked to Facebook, and Facebook did not offer an option to disable it (Jain & Ghanavati, 2020). Mounting criticism and legal action forced Facebook to disable Beacon, while some features that may cause privacy violations are still being developed by some companies, even though the invasion of privacy was not thought of at the time of development. Considering the reality of the situation, and the fact that protecting users’ privacy is not only a social responsibility, but also a legal obligation (GDPR, 2018a), this paper is going to mainly focus on three questions, which are:
“Why do the legal tools may fail to protect privacy?”,
“What factors affect privacy protection?”,
“What progress have been achieved and what is needed to be done in the future?”
Section 2.1. Why do the legal tools may fail to protect privacy?
Companies often collect data while users interact with digital products, which inevitably lead to potential data leakage problems. Hence, transparency and giving users control over their personal data is important, and companies should also be upfront with users about data collection practices, which is also explicitly stated in law (GDPR, 2018b). The artificial intelligent system is usually developed through a method called training, during which a large amount of data is required, just as teaching a child with textbooks. And continuous data collection could leads to an endless loop of data collection and threatens the privacy of users (Jain & Ghanavati, 2020). Siri, Google Assistant and Alexa, the three main virtual mobile assistants developed by three major giant technology companies (Apple, Google, Amazon), would store an ample amount of searching history and other data to improve products (Amazon Inc., 2023; Apple Inc., 2022b; Google Inc., 2022). In the era of Artificial Intelligence, it has become increasingly challenging for individuals to avoid utilizing digital platforms. At the same time, high-tech companies require user data to conduct research and development in various fields. For instance, search history is utilized to train machine learning algorithms, which provide personalized content recommendations (such as Google), while user requests are leveraged to enhance Natural Language Processing (NLP) capabilities. In 2019, Apple and Amazon were caught reviewing audio recordings of users without their knowledge or consent to improve their voice assistants, Siri and Alexa, respectively. The controversy arose as users' sensitive information was being accessed by real people without users’ consent. Authorities around the world have imposed legal obligations on businesses in relation to the handling of user privacy, in order to keep data safe from unauthorised access like the examples above. European Union (EU) emphasised in 1995 Data Protection Directive (95/46/EC, hereinafter called 1995 Act) , Section IV, Article 10 that data controllers shall provide the purposes of the processing for which the data are intended (95/46/EC, 1995) and in General Data Protection Regulation (GDPR), which aimed to replace the 1995 Act, EU stipulates data controllers shall take appropriate measures to provide information about how the users data was being collected, stored and processed (GDPR, 2018b); California, US marked out the right for the customers to know about the personal information a business collects about them and how it is used and shared (CCPA, 2018). Despite legal regulation imposed some obligations on corporations to protect user privacy, it is difficult to take every situation into consideration, thus, many companies, including Apple, who is famous for privacy protection, continue to collect data at the very margins of the law like applying some methods that were not clearly defined in law or taking the advantages of privilege clause (Wachter & Mittelstadt, 2018), which infers the idea that the current legal restriction is not enough to protect users’ privacy and the speed of legal iteration might be unable to keep up with the speed of technological updates. Unfortunately, since the current modern legislative system often requires a significant amount of time for the legislative process and the faster pace of development in the age of AI compared to before, the enforcement gap is likely to widen over time.
Meanwhile, the lack of resources may lead to failure in the application of legal means. On one hand, in cases where corporations are suspected of wrongdoing, investigations by authorities may be delayed or even indefinitely postponed due to a lack of personnel or resources to conduct a thorough review. Moreover, if a company chooses to employ various methods to circumvent judicial authorities, such as deliberately misleading investigators, more time and resources may be expended on the investigation process. Like Mark Zuckerberg did in 2018, during the several hours of the hearing, Zuckerberg deliberately admitted to some issues and intentionally avoided others in order to maintain the public image of the company and to delay the investigation process (Facebook, Social Media Privacy, and the Use and Abuse of Data, 2018). On the other hand, the complexity of technology in the era of AI could make the definition of illegal behaviour more challenging, since the AI system could possibly make decisions by itself, the responsibility can be hard to distinguish (Santoni de Sio & Mecacci, 2021).
Section 2.2. What factors affect privacy protection?
It’s widely accepted that governments play an important role in protecting citizens’ privacy, however, the authorities themselves sometimes threaten privacy protection. And governments could be the ones who are unwilling to see fully privacy protection, though it is not necessarily negative, but may have a role in maintaining national security. Following the 9/11 attacks, the U.S. government and airlines collected and stored passenger name records (PNRs) for the purpose of investigating the hijackings and evaluating the effectiveness of using PNR profiling to identify potential security threats. During the period of 2001 to 2003, many of the major airlines based in the US and various government agencies participated in these secret investigations without informing or obtaining consent from the individuals whose data were being used (Movius & Krup, 2009). In 2013, a former sub-contracted employee of US National Security Agency, Edward Joseph Snowden, reported to media that the US government have monitored thousands of users across the world by a project called PRISM. As reported, PRISM cooperated with 9 companies to collect and store user sensitive data to search for information (Kumar, 2017). Similarly, in Europe, the MI5, MI6 and GCHQ of UK also own programs to spy on people’s communication via smart devices (Edwards & Urquhart, 2015). Furthermore, whether intentionally or not, governments are attempting to weaken the secure and confidential nature of encrypted communications, according to a report by a United Nations department (OHCHR, 2022). Nevertheless, the paradox is that governments believe that encryption in any circumstances regardless of lawful and essential access to personal data like investigating crimes is non-responsible and even harmful to public security (UK Government et al., 2023), together with engagement with Communications Service Providers and monitoring of social platforms, government will have an enhanced capacity to fight terrorism (UK Government, 2018), but for the public, more surveillance may led to higher chances of potential misuse of personal information as well as leaking (Dinev et al., 2008). This view is supported by some of the authorities’ officials, like former U.S. Attorney General William Barr (2019) admitted that the inclusion of surveillance does degrade the security of the end-point user. Indeed, it is true that further research is needed to determine whether authorities’ surveillance can guarantee public security, the government's influence on privacy protection is clearly present.
Furthermore, different business models have varying implications for the needs of privacy information and consequently, the level of privacy protection that companies must implement. For instance, most of Facebook’s revenue is derived from advertising, making user data a valuable asset. However, for Apple, whose primary revenue relies on the sales of iPhones and iPads (Apple Inc., 2022a), user information may be not as crucial. Therefore, Apple is more willing to protect user privacy.
In addition to the factors of the companies, it is worth noting that users can also be a barrier to protecting privacy. To provide better services, many platforms directly ask the user for data access, during which the users give up privacy voluntarily. As previous mentioned in introduction section, most user think slightly of privacy policy, possibly resulting in the unknowingly obtaining of personal information (Ali et al., 2019). Meanwhile, users may choose to enter private information because the object of the conversation is AI. However, the database that AI uses is public and therefore can be accessed if using a specific method, which may lead to possible privacy leakage, as Shokri et al. (2021) concluded.
Section 2.3 What progress have been achieved and what is needed to be done in the future?
Since the development and application of AI involves a large amount of sensitive information such as health status, political beliefs, or financial situation, it is crucial to avoid direct linkage of specific data to the users in order to prevent potential privacy leakage; therefore, in practise, the sensitive information would be pre-processed by Differential Privacy methods before being analysed. The Differential Privacy methods include redaction, anonymization and pseudonymization which will be explained subsequent sections. But in short, de-identification removes identifying information from a dataset so that personal data cannot be associated with specific individuals (Garfinkel, 2015). However, the process does not fully guarantee the safety of the sensitive information. In 2008, two researchers from the University of Texas at Austin, Arvind Narayanan and Vitaly Shmatikov, published a paper entitled Robust De-anonymization of Large Sparse Datasets, detailing how privacy attacks could be carried out on data provided by Netflix. The researchers used a technique called link attack; by both analysing data from public database, IMDb, and the anonymised dataset provided by Netflix, the researchers successfully identified some anonymised users (Narayanan & Shmatikov, 2008). Although the research was conducted more than ten years ago and more effective de-deification methods were developed, a more recent study by Cheu and colleagues (2021) also demonstrated that it is possible to decrypt sensitive information from a dataset that has been processed by a Differential Privacy algorithm, which evidenced that even if the leaked data has been processed, it may still pose a risk of privacy breach. However, it does not mean that Differential Privacy is completely useless, the study also suggested that careful consideration and highlighted the critical role of efficient cryptographic techniques in mimicking central Differential Privacy mechanisms in distributed contexts can make the deployment of local differential privacy more secure, which will likely be the direction of future improvements.
Another practical technique is Federated Learning (FL), a burgeoning machine learning scheme that “leaves the training data distributed on the mobile devices, and learns a shared model by aggregating locally-computed updates” (McMahan et al., 2017, p. 1). FL was initially introduced by Google in 2017 as a Gboard (Google’s keyboard app) function to predict user’s text input, the significant difference from other machine learning is that FL allows mobile phones to work together and learn a prediction model collectively, while ensuring that all the training data remains on the respective devices. In addition to Google, other companies, such as Apple and Microsoft, have already applied this technology to products and made numerous improvements. Though it is believed that Federated Learning prevent sharing training data thus offering better privacy, a recent experiment showed that the local training data can be obtained from the shared information (Gradient) by a specific algorithm (Zhu et al., 2019). And another research even pointed out that “differential privacy possibly remains the only way to guarantee security” for successfully used similar method to obtain secured information (Geiping et al., 2020, p. 9). Therefore, further research should be conducted on whether federated learning can protect privacy and companies should be cautious when applying federated learning as well as other technology for there may also be potential security issues.
Apart from technology, the progress of the law is also remarkable since the implication of GDPR and CCPA provided fundamental provisions for privacy protection, although the existing legal structure does not fully ensure privacy security as previously explained in Section 2.1. Given this, one proposal is to empower individuals to challenge any unreasonable assumptions made about their data (Wachter & Mittelstadt, 2018), which gives autonomy to data subjects (users), enabling users to judge and dictate own will. Another suggestion is to improve the enforcement capacity. It is essential that enforcement agencies adequate resources and expertise to effectively apply the laws, including recruiting trained personnel and investing in technology as well as infrastructure to enable reliable monitoring.
Section 3. Conclusion
This paper concerns the common privacy and security challenges at the era of AI and find the possible solutions. The paper emphasizes that protecting user privacy is a social responsibility and a legal obligation in Section 2.1. However, legal tools may fail to protect privacy due to factors such as the complexity and rapid development of technology, and lack of resources during the law enforcement, which require improvement such as empowering individuals to challenge unreasonable assumptions about data and enhancing enforcement capacity.
In Section 2.2, the paper underscores the significance of government intervention, user consent, and business models in safeguarding privacy. The fact that authorities may at times engage in surveillance activities instead of protecting user privacy is surprising, which serves as a reminder that ensuring public security is not necessarily synonymous with strict privacy protection. Striking a balance between public security and individual privacy is crucial as well. Hence, Section 2.3 goes on from this point to elaborate on the current legal and technological solutions and limitations, suggesting that multi-faceted approaches are needed to achieve effective privacy protection in the era of AI.
This paper aims to provide a future direction for privacy protection strategies in the digital industry, however, as no practical experiments or tests have been conducted, the methods and recommendations mentioned in this paper are not guaranteed to be effective and are subject to further research.
References: 1 2 3 4 5 6 7 8 9 10 11 12 13 14 15 16 17 18 19 20 21 22 23 24 25 26 27 28 29
References
-
Ali, M. N. Y., Lizur, Md., & Jahan, I. (2019). Security and Privacy Awareness: A Survey for Smartphone User. International Journal of Advanced Computer Science and Applications, 10(9). https://doi.org/10.14569/IJACSA.2019.0100964 ↩
-
Amazon Inc. (2023, January 1). Privacy Policy—Amazon. https://www.amazon.com/gp/help/customer/display.html?nodeId=GX7NJQ4ZB8MHFRNJ ↩
-
Apple Inc. (2022a). Consolidated Financial Statements—2022 Q4. https://www.apple.com/newsroom/pdfs/FY22_Q4_Consolidated_Financial_Statements.pdf ↩
-
Apple Inc. (2022b, December 22). Legal—Apple Privacy Policy—Apple. Apple Legal. https://www.apple.com/legal/privacy/en-ww/ ↩
-
Barr, W. P. (2019, July 23). International conference on cyber security keynote. https://www.americanrhetoric.com/speeches/williambarrcybersecuritykeynote.htm ↩
-
Cambridge Dictionary. (2023). Artificial intelligence. In Cambridge Dictionary. Cambridge University Press. https://dictionary.cambridge.org/dictionary/english/artificial-intelligence ↩
-
Cheu, A., Smith, A., & Ullman, J. (2021). Manipulation Attacks in Local Differential Privacy. 2021 IEEE Symposium on Security and Privacy (SP), 883–900. https://doi.org/10.1109/SP40001.2021.00001 ↩
-
Dinev, T., Hart, P., & Mullen, M. R. (2008). Internet privacy concerns and beliefs about government surveillance – An empirical investigation. The Journal of Strategic Information Systems, 17(3), 214–233. https://doi.org/10.1016/j.jsis.2007.09.002 ↩
-
Edwards, L., & Urquhart, L. (2015). Privacy in Public Spaces: What Expectations of Privacy Do We Have in Social Media Intelligence? (SSRN Scholarly Paper No. 2702426). https://doi.org/10.2139/ssrn.2702426 ↩
-
Facebook, social media privacy, and the use and abuse of data (S.Hrg. 115-683). (2018). U.S. GOVERNMENT PUBLISHING OFFICE. https://www.govinfo.gov/content/pkg/CHRG-115shrg37801/html/CHRG-115shrg37801.htm ↩
-
Garfinkel, S. L. (2015). De-identification of personal information (NIST IR 8053; p. NIST IR 8053). National Institute of Standards and Technology. https://doi.org/10.6028/NIST.IR.8053 ↩
-
GDPR. (2018a, November 14). Art. 1 GDPR - Subject-matter and objectives. GDPR.Eu. https://gdpr.eu/article-1-subject-matter-and-objectives-overview/ ↩
-
GDPR. (2018b, November 14). Art. 12 GDPR - Transparent information, communication and modalities for the exercise of the rights of the data subject. GDPR.Eu. https://gdpr.eu/article-12-how-controllers-should-provide-personal-data-to-the-subject/ ↩
-
Geiping, J., Bauermeister, H., Dröge, H., & Moeller, M. (2020). Inverting Gradients—How easy is it to break privacy in federated learning? Advances in Neural Information Processing Systems, 33, 16937–16947. https://proceedings.neurips.cc/paper/2020/hash/c4ede56bbd98819ae6112b20ac6bf145-Abstract.html ↩
-
Google Inc. (2022, December 15). Privacy Policy – Privacy & Terms – Google. https://policies.google.com/privacy?hl=en-US ↩
-
Jain, V., & Ghanavati, S. (2020). Is It Possible to Preserve Privacy in the Age of AI? Proceedings of the PrivateNLP 2020: Workshop on Privacy in Natural Language Processing, Vol-2573, 32–36. https://ceur-ws.org/Vol-2573/PrivateNLP_Paper4.pdf ↩
-
Kumar, P. (2017). Corporate Privacy Policy Changes during PRISM and the Rise of Surveillance Capitalism. Media and Communication, 5(1), 63–75. https://doi.org/10.17645/mac.v5i1.813 ↩
-
Ma, C., Li, J., Ding, M., Yang, H. H., Shu, F., Quek, T. Q. S., & Poor, H. V. (2020). On Safeguarding Privacy and Security in the Framework of Federated Learning. IEEE Network, 34(4), 242–248. https://doi.org/10.1109/MNET.001.1900506 ↩
-
McMahan, B., Moore, E., Ramage, D., Hampson, S., & Arcas, B. A. y. (2017). Communication-Efficient Learning of Deep Networks from Decentralized Data. Proceedings of the 20th International Conference on Artificial Intelligence and Statistics, 1273–1282. https://proceedings.mlr.press/v54/mcmahan17a.html ↩
-
Movius, L. B., & Krup, N. (2009). US and EU privacy policy: Comparison of regulatory approaches. International Journal of Communication, 3, 19. ↩
-
Narayanan, A., & Shmatikov, V. (2008). Robust De-anonymization of Large Sparse Datasets. 2008 IEEE Symposium on Security and Privacy (Sp 2008), 111–125. https://doi.org/10.1109/SP.2008.33 ↩
-
OHCHR. (2022). The right to privacy in the digital age. https://documents-dds-ny.un.org/doc/UNDOC/GEN/G22/442/29/PDF/G2244229.pdf?OpenElement ↩
-
Santoni de Sio, F., & Mecacci, G. (2021). Four Responsibility Gaps with Artificial Intelligence: Why they Matter and How to Address them. Philosophy & Technology, 34(4), 1057–1084. https://doi.org/10.1007/s13347-021-00450-x ↩
-
Shokri, R., Strobel, M., & Zick, Y. (2021). On the Privacy Risks of Model Explanations. Proceedings of the 2021 AAAI/ACM Conference on AI, Ethics, and Society, 231–241. https://doi.org/10.1145/3461702.3462533 ↩
-
TITLE 1.81.5. California Consumer Privacy Act of 2018 [1798.100—1798.199.100]. (2018). https://oag.ca.gov/privacy/ccpa ↩
-
UK Government. (2018). The United Kingdom's Strategy for Countering Terrorism. https://assets.publishing.service.gov.uk/government/uploads/system/uploads/attachment_data/file/716907/140618_CCS207_CCS0218929798-1_CONTEST_3.0_WEB.pdf ↩
-
UK Government, US Government, Japan Government, & Australia Government. (2023, January 16). International statement: End-to-end encryption and public safety (accessible version). GOV.UK. https://www.gov.uk/government/publications/international-statement-end-to-end-encryption-and-public-safety/international-statement-end-to-end-encryption-and-public-safety-accessible-version ↩
-
Wachter, S., & Mittelstadt, B. (2018). A Right to Reasonable Inferences: Re-Thinking Data Protection Law in the Age of Big Data and AI [Preprint]. LawArXiv. https://doi.org/10.31228/osf.io/mu2kf ↩
-
Zhu, L., Liu, Z., & Han, S. (2019). Deep Leakage from Gradients (arXiv:1906.08935). arXiv. http://arxiv.org/abs/1906.08935 ↩